We do love our cars. “Made in Germany” represents sophisticated engineering, innovation, and over 100 years of solid manufacturing experience; Germany’s automotive excellency has even attracted tech giant, Tesla, to establish their automotive manufacturing base here and bask a little in the light.
And yet it seems that this solid manufacturing experience does not stand traditional manufacturers in good stead when dealing with the current volatile market. Otherwise why are so many established car makers still waiting on parts while Tesla reports record vehicle sales?
Shortages of semiconductor chips for example, are estimated to have cost car makers $210 billion in 2021. The current, unprecedented challenges to global supply chains are, as Deloitte reports, are now causing established automotive and industrial companies to “take a long hard look at resiliency”.
Established car makers can learn from the faster-moving tech industry: Advanced data science can help digitally-enabled manufacturers build resilience and minimize the impact of disruption.
Conventional industry methods are unable to handle the increasing complexity that accompanies big data. Data science techniques, on the other hand, are able to deftly capture, process, and analyze the big data typical of the manufacturing industry.
Data science for smart analysis and accurate predictions
As revealed by 2021 research into supply chain disruptions and resilience, the industry can benefit from the “positive impact of big data analytics and machine learning for real-time supply-chain and decision-making models”, for “data promptness and accuracy… transparency, traceability, and flexibility”.
With a data science tool, manufacturers can access and integrate huge amounts of data of different types and sources into their analysis: from supplier behavior, product demand, customer behavior, consumption of inventory items, even through to volatile, external influences such as shifting geopolitical factors impacting supplier locations.
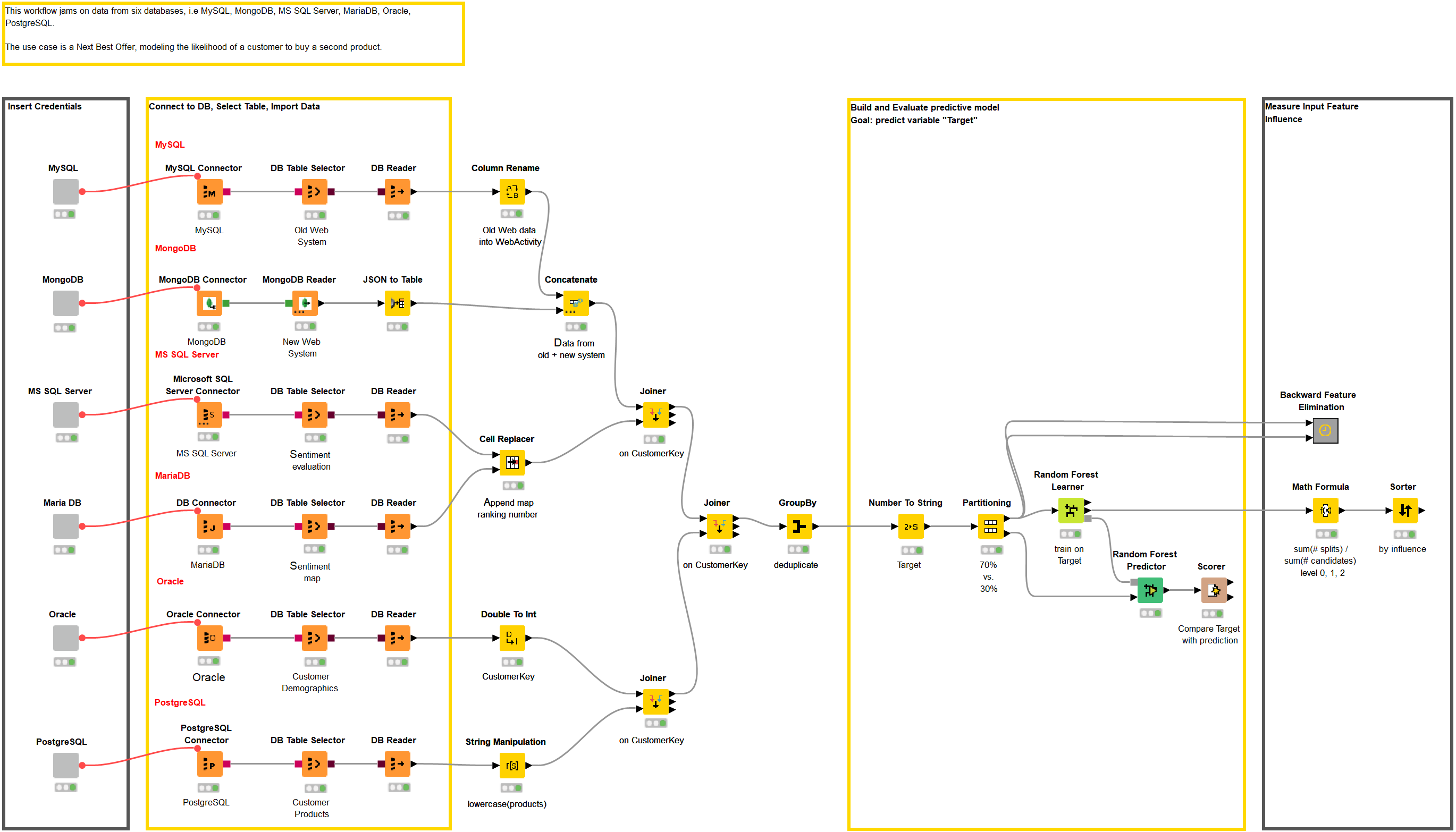
Manufacturing operations such as inventory-level management, logistics routing, production line downtime, capacity planning — to name only a few — can all benefit from the more accurate analysis available through big-data-compatible data science techniques.
Tip: Find hands-on solutions with KNIME for Manufacturing on the KNIME Hub
With easy access to the full data, the true complexity of the situation can be analyzed and more accurately predicted. Companies can use their improved analysis of the status quo to produce smart predictions of the future. This has the potential to enable more accurate inventory-level management, resource allocation, better logistics routing, maintenance planning, improved forecasting of future product demand, customer engagement, market behavior…happily the list is long.
This additional insight will enable manufacturers to see more clearly where efficiency gains might actually pose a risk (the low cost option could be a fragile option) and then strike an optimal balance – increasing the resilience of the process.
A case in point: AI-based inventory optimization for resilient warehouses
To keep warehouses filled sufficiently, inventory managers need to understand the behavior of diverse variables. When stock levels get low it’s often because incorrect assumptions were made about demand.
For a good trade-off between inventory costs, fragility of supplies, and stock levels, inventory managers can use data science techniques to predict expected supply and consumption levels.
To produce these accurate predictions amidst increasing complexity, manufacturers need a tool that is able to process and blend large volumes of unstructured data from disparate sources.
The advantage of Inventory Level Optimization with a data science tool such as KNIME is that huge amounts of data can be read in and processed without exhausting compute-resources. With automotive warehouses stocking literally thousands of components, this is a basic requirement. After processing the data, the data science solution applies time series analysis: The solution learns in real time from the changing data, immediately turns the new data into insight, and adjusts the prediction of future consumption.
The results of the analysis can be provided to the inventory managers as an analytical service in a browser-based data app. The analysis is presented in a suitable format for the manager to consume easily, check stock levels, and write back the calculated order plan to the supply chain management system, ensuring optimal inventory levels.
A case in point: Data science for resilient production lines
Any shortage of workers on the production line poses a risk to meeting delivery deadlines. Sadly, automotive not only suffers from a lack of skilled workers but is also one of the most turnover-prone industries. An 2019 industry report found that two out of five manufacturers suffer turnover rates of over 20%.
In the short term, car makers can improve their resilience to workforce disruptions with more efficient operator capacity planning and employee attrition prediction.
In the longer term, automotive can improve resiliency by addressing high turnover rates and talent gaps with upskilling programs.
Predict operator capacity, improve planning, increase resilience
Line management is vulnerable because workforce shortages are anticipated using experience-led methods rather than being accurately predicted by data-driven techniques. This makes the planning process overly complicated, time consuming, and error prone. With a more detailed, accurate, and up-to-date picture of plant operator resources, the production line could be manned resiliently.
Using a data science solution, line managers can predict operator capacity with 96% accuracy. This makes planning much more efficient and line managers no longer have to worry they won’t make delivery deadlines.
Predict employee attrition, upskill workers, improve retention
With a similar data science solution, HR data can be used to predict when employees will leave the company. The prediction results can be sent to HR on a regular basis enabling the HR manager to retain employees by intervening in good time, improving retention programs, and ultimately helping ensure a robust production line.
Upskilling programs can “improve employee engagement and retention and attract new talent” says Forbes. By empowering employees to develop a broader skill set, the workforce becomes more agile and the company more adaptable — more resilient — to market change.
Enabling workers to expand their skills to include data analytics will increase process efficiency. Typical for automotive are the time-consuming data analysis bottlenecks that occur when analysis always has to go through IT.
By giving employees an intuitive, visual programming data science tool that is easily learned, access to data analytics is enabled at both a general and expert level throughout the company. With such a low-code tool, no previous programming knowledge is required. Workers can be upskilled to even build the analysis and predictive models described in this article themselves, reducing data analysis bottlenecks.
A Volatile Market Countered with (Calm) Data Science
Industry 5.0 encourages manufacturers to revise their existing practices and leverage technology to streamline their production processes, and build in resiliency.
Resilience to the rapid changes in today’s market behavior can be achieved by adopting a flexible and agile data science solution. Data science techniques give companies the means to process huge volumes of data easily, analyze the industry’s diverse variables automatically, and respond to change quickly.
The subsequent ability to predict calmly and accurately how the highly volatile elements of the market might behave in the future will enable companies to make clever trade-offs: Instead of focusing solely on efficiency gains — which could lower resilience — car makers will have a more accurate analysis at their fingertips and can move from vulnerable to resilient manufacturing.