Manufacturers are stuck. If they’re not stuck in old ways of working, they’re stuck in what McKinsey calls “pilot purgatory” – where critical, innovative use cases do not advance beyond the pilot stage to full-scale rollout.
And yet, the same report emphasizes that manufacturers demonstrate a significant willingness to identify and adopt performance-improving technologies. Data science in Manufacturing in particular offers a range of ways to transform the production value chain, increasing operational efficiency and maximizing returns.
The question is: How can manufacturers get unstuck and implement these methods? A question which gains ever more urgency given the need for manufacturers to innovate and meet the severe challenges of the future.
Over the past few months, KNIME conducted a deep-dive into how manufacturing can better leverage data science to advance beyond the pilot stage and lift-off into operationalization. Here are 6 of the ways we identified.
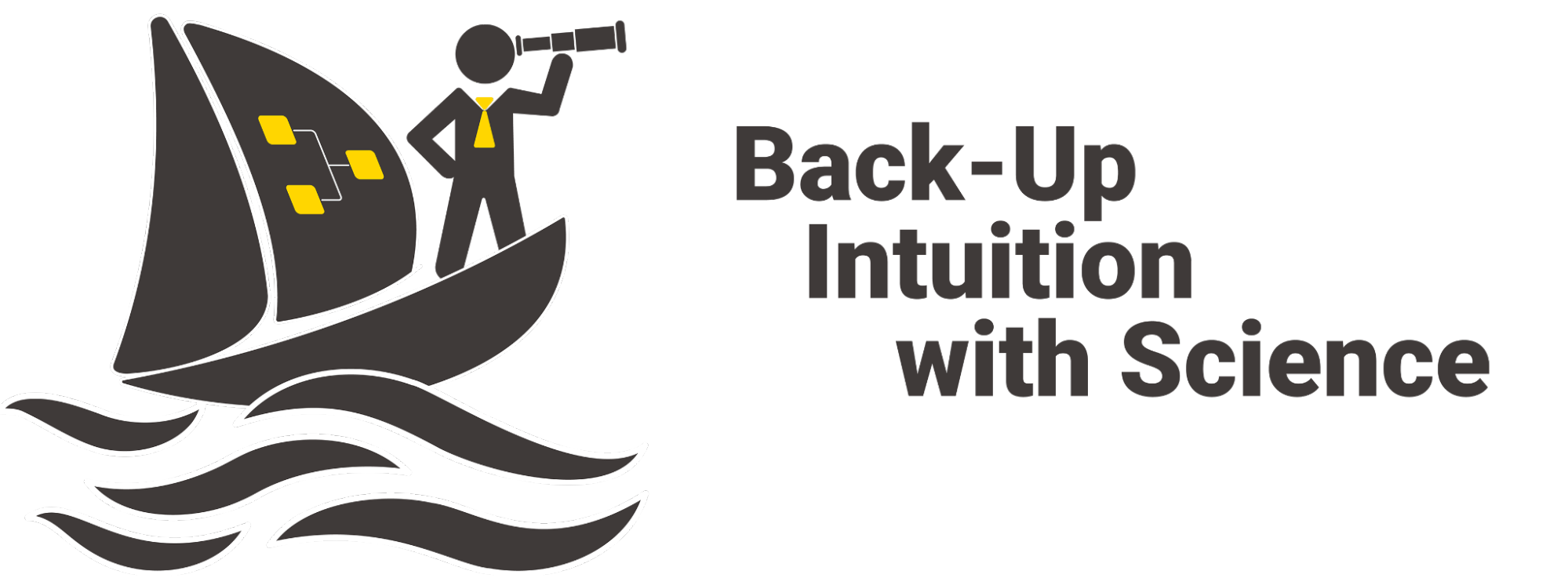
There’s a time and a place for intuition, based on factory-floor experience. Capacity planning, for instance, is not the time and place. Too many decisions are still being made based on managers’ hunches and subjective experiences.
So, how can data science provide a more reliable solution? By redressing the core weakness in workforce planning. Much of capacity planning is already data-driven, but when it comes to planning for the number of plant operators, experience is relied upon. Needless to say, this is a highly error-prone approach.
Instead, a plant’s data can be used to build an operator capacity plan. When predictive analytics are applied to the data, planners get the insight to optimize operator capacities ahead of events, bringing real benefits in time- and cost-savings.
Using KNIME Analytics Platform, a capacity planning predictor was set up to test this idea. It returned an accuracy score (R²) score of 92%: An approach with far more reliability than experience alone.
Read a walkthrough of how the team built a predictor for operator capacity planning.
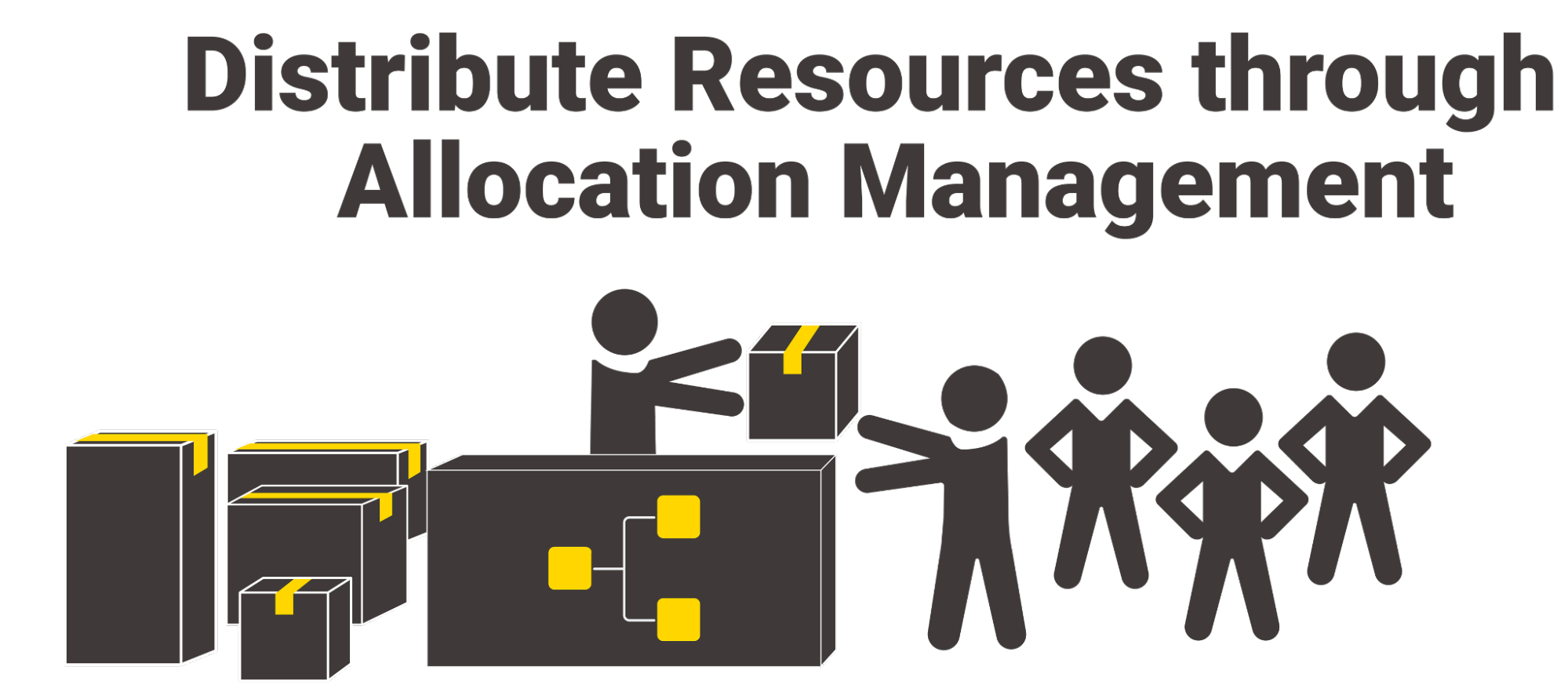
Many of the digital processes in widespread use across manufacturing are designed for normal times: Times of sufficient supply.
But during global shocks like today’s semiconductor shortage, you cannot fulfill every customer demand. Supply-chain disruptions, capacity container problems and component shortages can all lead to supply-side challenges. The Covid-19 pandemic has exacerbated all three, leading to global order volumes falling by 24 points in Q3 2021.
Hence, smart data analytics solutions can fill the gap in Enterprise Resource Planning (ERP). Manufacturers need to decide: Will we effectively run into a shortage of a particular part, or can we survive by sending small but marginally sufficient quantities to respective plants?
If a shortage appears unavoidable, Resource Allocation Management needs to decide which facility and ultimately which customer receives how much of a short raw material. Such planning can help make distribution more reasonable for all businesses, and alleviate panic-buying and stockpiling by more powerful players. Uncertain futures make the need to roll-out Allocation Management plans all the more necessary.
Read an interview with Arne Beckhaus, Head of Data Services at Continental Engineering Services, on using data science in Resource Allocation Management.
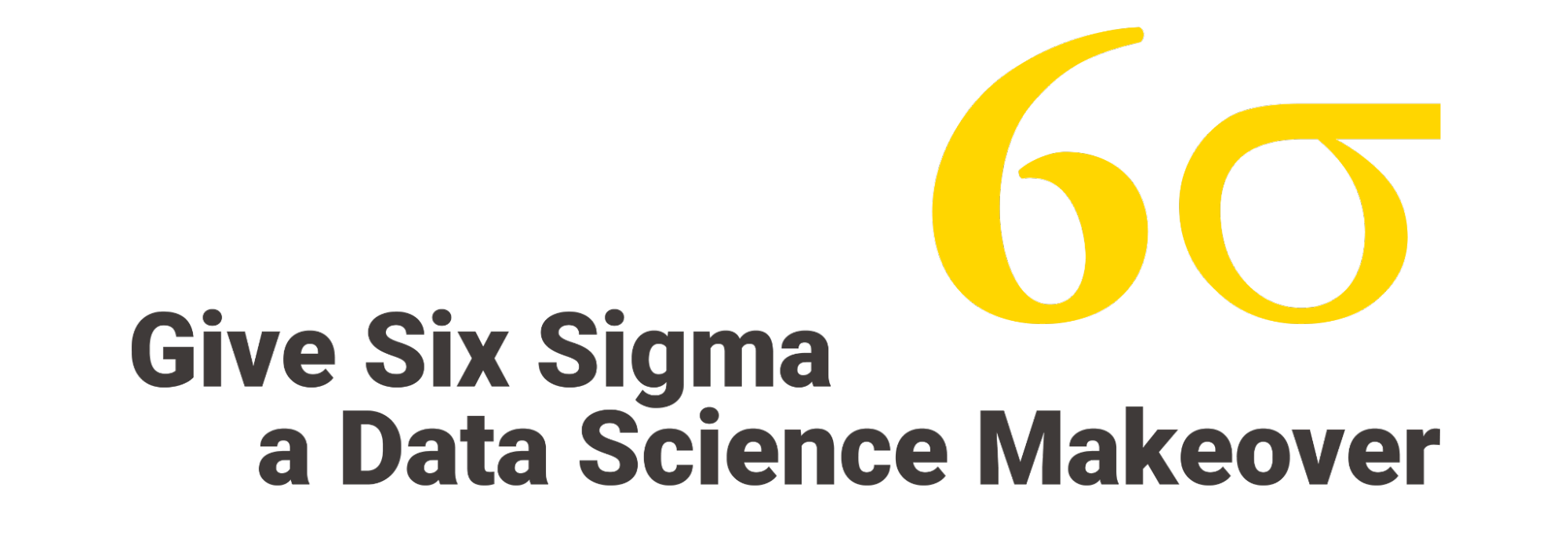
It’s not just technologies and old methods which could do with revision: Entire manufacturing production processes can be thoroughly reorganized. Take the Six Sigma model, for instance. This set of strategies — designed to improve business processes — is used by 53 percent of Fortune 500 companies.
It's not surprising that in the wake of the upheaval to manufacturing processes following the COVID-19 pandemic, the expertise of these process improvement experts is sought-after. And yet, the Six Sigma master blackbelts are having to rethink too.
Working through the stages of their DMAIC projects (Design, Measure, Analyze, Improve Control), the problem Six Sigma experts find is that DMAIC does not iterate back to the very beginning and collect new data.
In order to provide a truly sustainable process they need to merge their analysis and control phases and create an iterative cycle. By integrating a data science approach they can not only monitor process performance but reevaluate and re-operationalize as required.
Read a walkthrough of how a team integrated data science into a DMAIC project to reduce chip formation and improve error recognition.
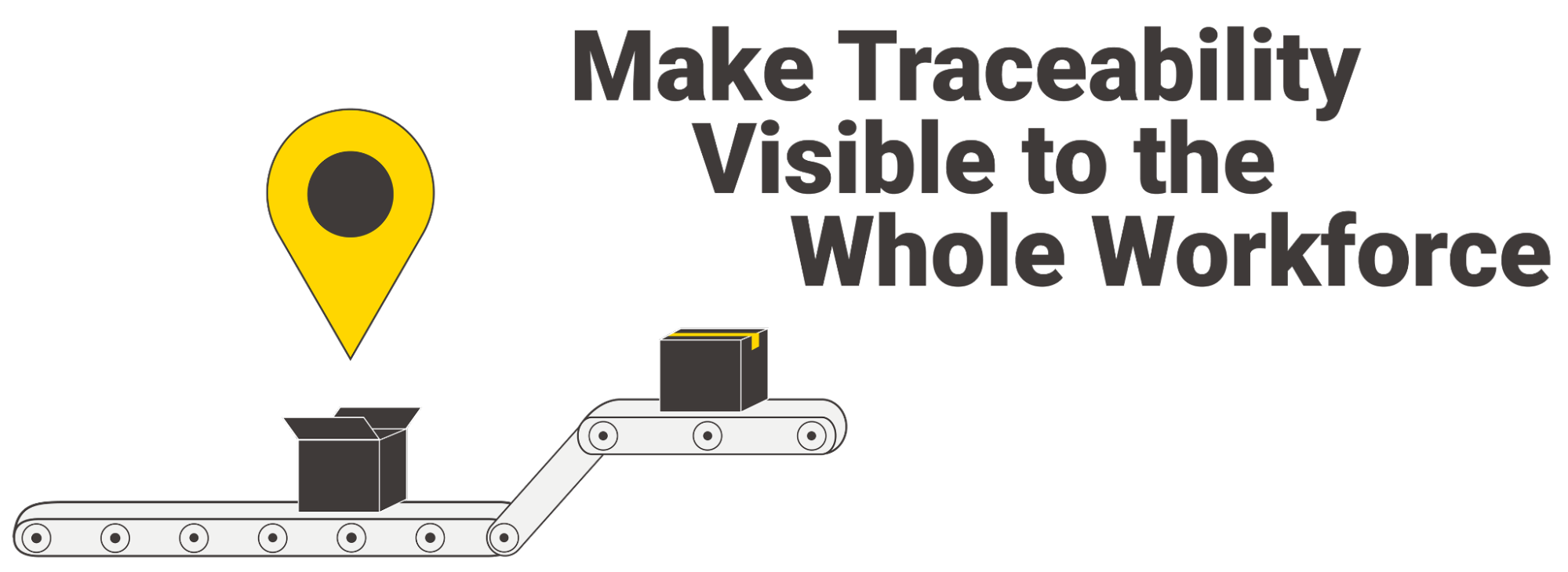
A small commercial aircraft typically contains 4 million parts made by 1,500 companies: Every single one of these parts needs to be high quality and functional. This is why traceability is so vital: It makes it possible to track raw materials, parts, and goods through a manufacturing process using data.
By using shop-floor IT systems to record the serial or batch identifier of components throughout the production process, manufacturers can generate large volumes of traceability data. This data provides a valuable digital mechanism for a thorough understanding of the production process, as well as offering quality control, and management and debugging of complaints.
However, some manufacturers still keep this data as the preserve of a few IT experts within the organization. This is a problem, as traceability is most effective when it is accessible to everyone throughout a business. When business users have access to a single interface where they can access and use this product data, they become independent and IT stops being a bottleneck.
If a part is found to be faulty, a product recall might need to be performed. If all workers can see which parts are faulty in a single, usable interface, manufacturers give themselves the best chance of putting out that fire quickly.
Read how Continental embarked on the data literacy journey and uses KNIME as a self service tool for traceability to provide answers in minutes rather than days.
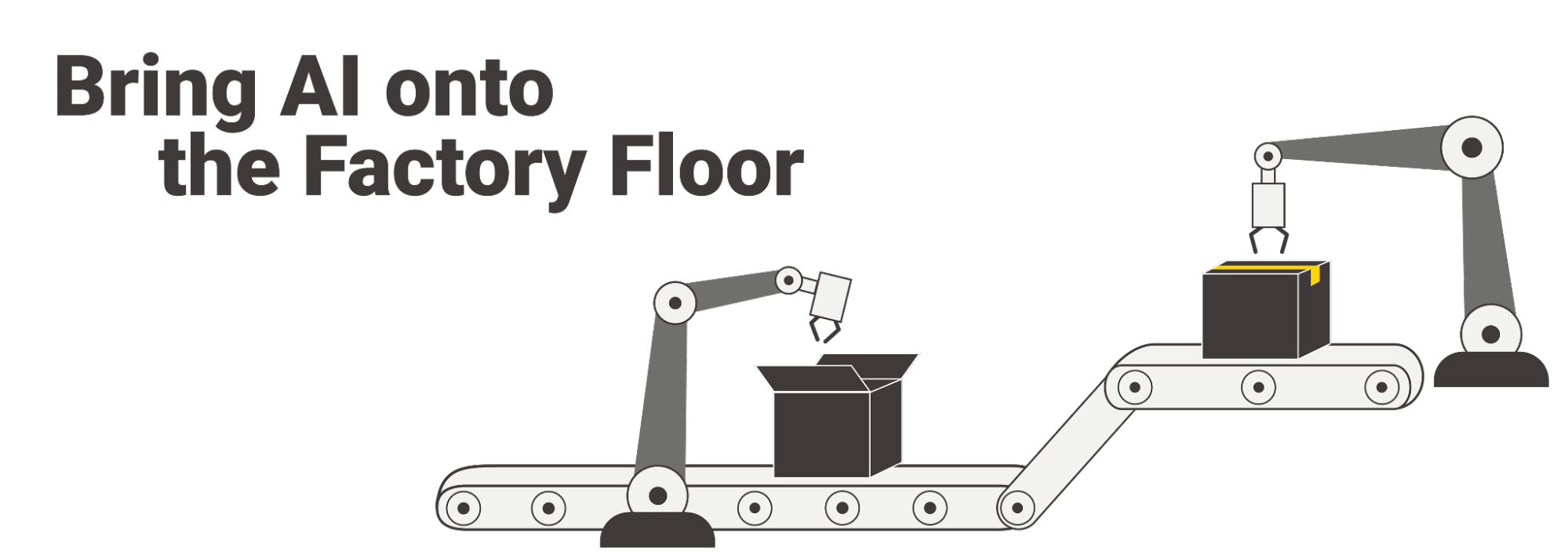
While “93% of companies believe that AI will be pivotal in driving growth and innovation in the manufacturing sector”, actual tool adoption is slow , according to Deloitte.
Although many tasks on the factory floor require interaction with data, such as signal monitoring, the truth is that many floors are decades behind fully benefiting from cutting-edge AI and data science. This has far-reaching implications for business, with non-smart factories being less safe, more wasteful of resources, and offering less time-to-value for their products.
So why, in general, are AI and Data Science not being brought onto the floor? One major reason has been the lack of a common operational language between manufacturing and data experts. Now, however, both groups can use the same low-code tool: Data experts put together an interface through which manufacturing experts interact.
Modern data science environments allow automation and interaction to be combined flexibly so everyone in a company can benefit from AI and machine learning. In a real-world setting, this would mean manufacturing experts could use their experience to steer production through the application interface. They could override the decision of the data model, assess the quality of the model, give additional feedback to the model, so it could become more accurate over time.
Read a discussion of AI in manufacturing and how data science can be better integrated on the factory floor.
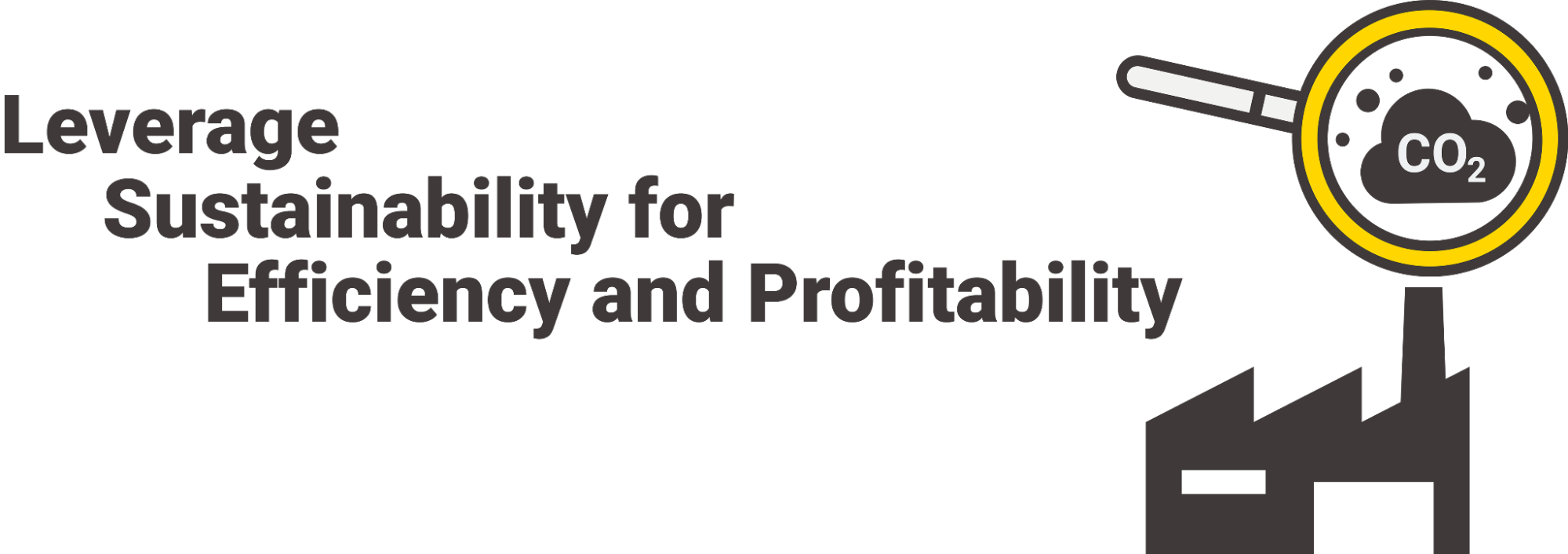
According to a World Economic Forum survey “66% of all respondents, and 75% of millennial respondents, said they consider sustainability when making a purchase”. Without doubt, consumers want sustainable futures, and for those, sustainable industries.
Reducing the carbon footprint of products requires a deep understanding of their components. In the Life Cycle Assessment (LCA) model, various data (for e.g., data about the carbon footprint of components from raw material extraction right through to production) are processed and analyzed. If components have high carbon footprints, alternatives can be sourced to reduce a product’s overall footprint.
But as current LCA methods are costly, and require manual data-handling, scaling becomes impractical and unrealistic - especially for manufacturers with large product portfolios. New concepts and models are needed: Built on KNIME’s own Data Science Life Cycle (DSLC), the Sustainability-DSLC is a way of analyzing the environmental hotspots of thousands of individual product components quickly. When a hotspot is identified, sustainability experts can source alternatives, and reduce the product’s carbon footprint.
Developed by the Fraunhofer Institute and the University of Stuttgart, the model gives sustainability experts the ability to make business more profitable through eco-friendly means.
Read how Daniel Wehner, researcher at Fraunhofer IBP, and his team came up with the S-DSLC for sustainable manufacturing.
Data Science in Manufacturing
There are many more ways in which Data Science in Manufacturing can transform the production value chain and help manufacturers get unstuck. Getting out of pilot purgatory requires careful planning and investment, but once achieved, dramatically alters the potential for new possibilities: From reactive firefighting, right across to proactive decisions on sustainability and workforce planning.