Life Cycle Assessment is manufacturing’s secret weapon for sustainability. Unfortunately, it’s misfiring.
Reducing the carbon footprint of products requires a deep understanding of their components. In the Life Cycle Assessment (LCA) model, various data (for e.g., data about the carbon footprint of components from raw material extraction right through to production) are processed and analyzed. If components have high carbon footprints, alternatives can be sourced to reduce a product’s overall footprint.
But as current LCA methods are costly, and require manual data-handling, scaling becomes impractical and unrealistic — especially for manufacturers with large product portfolios.
Additionally, sustainability experts can be easily overburdened by the sheer number of products and business processes requiring analysis. As such, involving an expert for every individual problem is simply not feasible. The result: Managers do not receive the information they need to make wide-ranging, sustainability-driven decisions.
Daniel Wehner, a researcher based in Germany’s Fraunhofer Institute for Building Physics (IBP) argues for a new data model to make sustainable manufacturing a reality:
“What the existing method of LCA of products does well is developing understanding of the optimization potentials and environmental drawbacks — or ‘hotspots’ — of individual products and processes. However, the method is cost-intensive as it involves manual data engineering — and even then, it can only work with the existing data businesses have for their products.”
He adds: “This is why we came up with the Sustainability Data Science Life Cycle.”
The S-DSLC: Automating workflows for scalability and sustainability
The Sustainability Data Science Life Cycle (S-DSLC) is a model for automating Life Cycle Assessment (LCA) workflows for large product portfolios. It thus eliminates a bottleneck associated with traditional LCA analysis.
In the S-DSLC model, a manufacturing company creates an automated workflow to quickly assess the life cycle of large numbers of components. Product data is prepared by sustainability experts and fed into the workflow. The results of the automated analysis are delivered to the relevant business experts through a tailored interface.
The business experts use this data to make sustainability decisions, as well as validate the model with their own product data. Their validating information aids maintenance of the model through updates and fixes. Overall, this helps the manufacturer achieve greater sustainability and as such remain competitive.
Developed by Wehner and colleagues from the Fraunhofer IBP and University of Stuttgart, the S-DSLC is integrative, as it builds upon frameworks from several data science models. It combines the LCA framework with the cross-industry standard process for data mining (CRISP-DM), and the Data Science Life Cycle (DSLC; developed by KNIME).
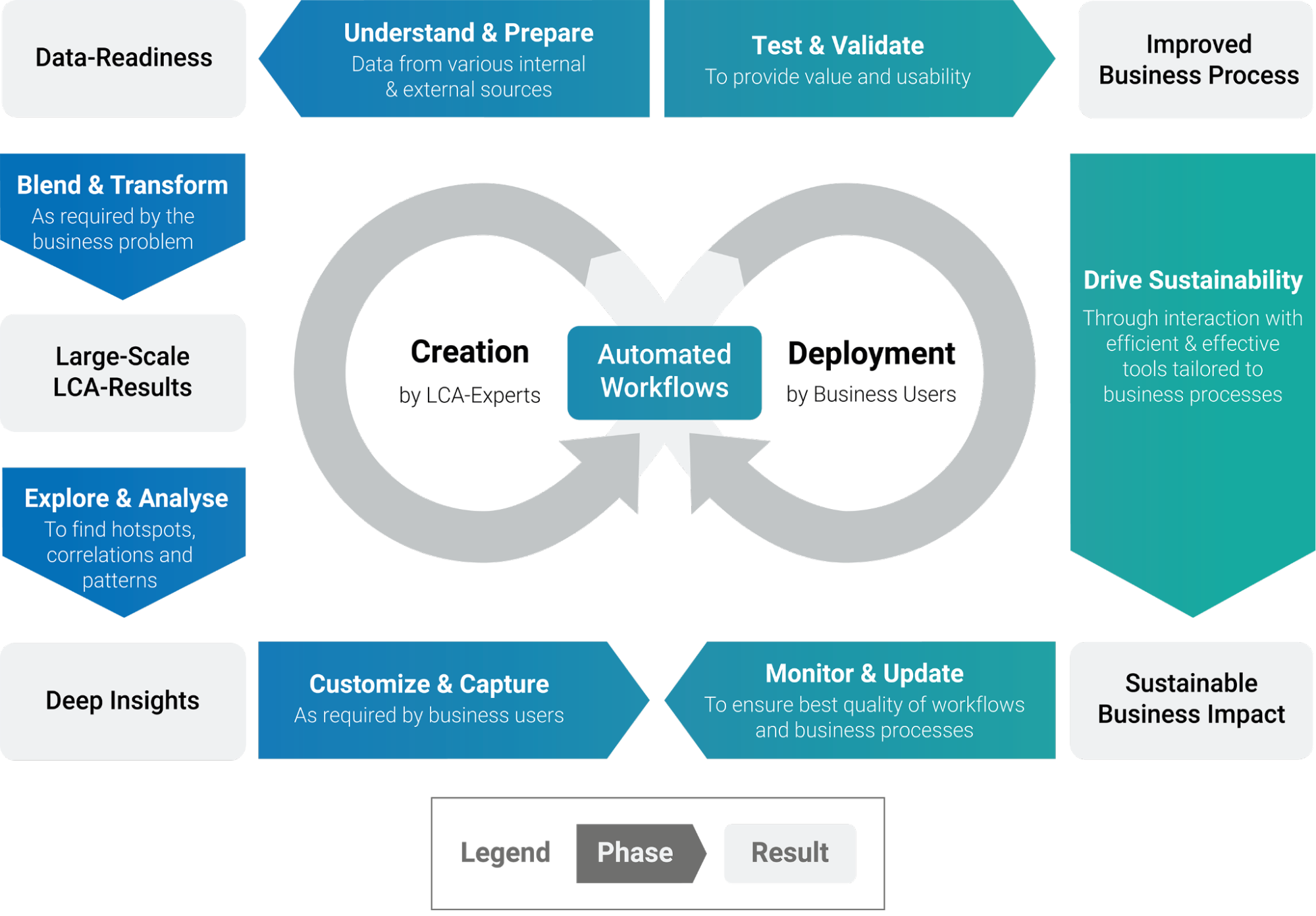
“The model is designed with data creation and productionization in mind,” says Wehner. “The Data Science Life Cycle already focuses on productionization, or how data is consumed by users. This S-DSLC goes a few steps further and adds sustainability as a goal, through its inclusion of automating LCA workflows. So, it’s designed with a focus on productionizing product life-cycle data, so it can be used to make sustainability decisions.”
Automation and scalability: A huge multiplier effect for sustainability
The potential for the S-DSLC model to be scaled across businesses with large product portfolios is immense. Automating LCA workflows reduces costs significantly, making it relatively affordable to capture sustainability insights for a large portfolio of products, and deliver those insights across an organization.
No longer would sustainability experts be forced to painstakingly prepare LCA reports for a small number of products to help managers in their decision making: The S-DSLC structures how the work can be done quickly for thousands of products.
Wehner adds: “Essentially, it gets the right data to the relevant decision makers automatically and empowers people without sustainability and data science expertise to make use of the data for evidence-based decisions. The decision makers can choose to find alternative providers in the supply chain, if a component — say a wire — is found to have a high carbon footprint. Now, imagine that for tens of thousands of components, which make up several hundred products, all done quickly. The multiplier effect for sustainability is huge.”
Automating LCA workflows has other tangible operational benefits. It frees up sustainability and data experts to work on more challenging things, giving decision makers the information they need to make sound sustainability choices.
Wehner emphasizes this point: “If you have a small team of sustainability and data experts in your manufacturing firm, you most likely want to use them to do something high level. LCA analysis of individual products is not exactly the best use of a data expert’s time. That’s why the S-DSLC model is so effective. It’s designed to help sustainability managers and others make decisions about large numbers of products and components without data science expertise. It’s designed to help real manufacturing businesses achieve sustainability.”
Upskilling for organization-wide data literacy
Although the S-DSLC is designed to make sure non-experts can use the information that the workflow delivers to them, it’s imperative that the workforce is attuned to this kind of information so they can work autonomously. “It’s not ideal if the workforce is then asking the data and sustainability experts what the data means, even after it’s been processed”, says Wehner. “Data and sustainability experts should monitor all workflows to help business experts, but upskilling the workforce may be needed”.
As such, the implementation of the S-DSLC model is an ideal opportunity for manufacturers to provide training to their workforce and upskill them. In the context of manufacturing, researchers from Cambridge, Exeter and Cranfield universities write that: “…Innovation alone is not sufficient to achieve sustainable intelligent manufacturing…It also requires the design of new, data-driven business models…and business ecosystems to facilitate this transition.”
The “new business ecosystem” includes upskilling of the workforce. But facilitation of new skills isn’t just a manufacturing challenge: It’s global. This is a point which the World Economic Forum hammered home in a recent report: “To prevent an undesirable lose-lose scenario — technological change accompanied by talent shortages, mass unemployment, and growing inequality — it is critical that businesses take an active role in supporting their existing workforces through reskilling and upskilling, that individuals take a proactive approach to their own lifelong learning.”
To ensure that they remain competitive by capitalizing on the strategic advantages that sustainability offers, it’s necessary that manufacturers implement methods like the S-DSLC. And to make the best use of those models, non-expert workforce will benefit from even basic data upskilling.
Sustainability, finally just makes sense for business
A recent survey of consumer attitudes to sustainability from the World Economic Forum found that “66% of all respondents, and 75% of millennial respondents, said they consider sustainability when making a purchase". Without doubt, consumers want sustainable futures, and for those, sustainable industries. Needless to say, un-sustainably produced products will affect consumers’ purchasing decisions, and we could be looking at a future where there simply isn’t a market for un-sustainable manufacturing.
But there are also other sound business reasons for prioritizing sustainability:
-
Optimized supply and production chains reduce waste and help make businesses more efficient.
-
The strategic advantages gained by more sustainable practices across these chains accrue — especially when employing a life-cycle framework — to make a business increasingly competitive, says the Harvard Business Review.
-
Sustainability-oriented practices can help meet the requirements of regulations and wishes of investors, both of which demand that products have reduced carbon footprints.
-
When scaled across business departments and locations, data-driven sustainability practices can encourage workforce upskilling, making departments more independent.
“Sustainable business practices” have made significant inroads into the growth agenda of forward-thinking CEOs, as Accenture highlights in their latest global CEO Study. Nevertheless, manufacturers still face one significant challenge when implementing sustainable processes across their businesses: Scaling, particularly the scaling of sustainability analysis of products. The S-DSLC is a model which helps manufacturers achieve sustainability at scale, and in doing so, helps them to remain competitive.
With the model developed, ecological and business arguments in favor, and encouragement from all angles to upskill their workforce, the only thing left to say is: When it comes to sustainability, manufacturers really have no excuses.