The future has come and gone, and our factories are still not very smart.
Walking down the factory floor, it’s true that you’d see many people making decisions based on data. You’d find, for example, control room operators monitoring signals on screens to make judgment calls about when to take action. You’d also find operators performing diagnostics tests on various pieces of equipment. They’d interpret charts to know when it’s time to troubleshoot. But this technology is over 30 years old.
The harsh reality is that the majority of factory floors are decades behind fully benefiting from cutting-edge data science. Rarely will you find advanced methods to do things like predictive maintenance, sustainable manufacturing, and algorithmic allocation management. These initiatives tend to live and die as pilot projects in what McKinsey calls “pilot purgatory”.
In today’s world however, the consequences for not adopting advanced analytics and data science are far-reaching. Beyond business concerns, like reducing inefficiency and growing the bottom line, manufacturers are held accountable to addressing more human ones. These have tangible benefits:
-
Smart factories reduce the chance of risk and injury to employees.
-
Reducing waste helps plants meet the demands of a sustainable future.
-
Improving time-to-value helps produce valuable and life-saving products, like Covid vaccines.
What’s more, not meeting these demands poses an existential threat to manufacturers.
So what’s the holdup? When it comes down to it, it’s people, not computers.
Today’s Data-Rich, Communication-Poor Process
Most facilities have the sensors to collect a ton of data. Most also tend to have the IT infrastructure to store and protect that data. And most hire teams of data experts sitting in Centers of Excellence, who are exceptionally trained in applying sophisticated algorithms to business problems. Where things start to break down is in the undefined process between how data experts and manufacturing experts work together.
Data teams typically involve a subject matter expert in the beginning and at the end of their project. In this case, we’re talking about a manufacturing expert, typically someone who knows the equipment, or the product in question.
The data expert begins by trying to understand the business problem. Let’s say, for example, the business would like to know exactly when a machine part will wear out, so they can replace it before it breaks. This will decrease costs and increase predictability for when that part should be ordered.
The data experts start by working with technicians and manufacturing managers to understand all the signs of wear in the machine. After understanding the current approach to the problem, the team starts on the most challenging task: finding and collecting all the necessary data. At this point, already, many projects fail. The team deals with legacy systems, different file formats, offline systems that, in many cases, are not accessible. The task of data blending is 90% of a data expert’s work.
If they manage actually gathering the data that they need, then the data experts then try to figure out a formula for detecting the damage that includes a multitude of variables. Finding and weighing these variables either through a human-devised formula or through a Machine-Learning devised formula is their first and foremost responsibility.
This hard work can take weeks or months, depending on the complexity of the solution.
Once they’ve built a model, one of three things can happen:
-
The manufacturing expert might say the data experts did it wrong. No, the temperature of the lab is not a significant factor and the model is going to make very expensive (and incorrect) recommendations. This means it’s back to the drawing board for the data experts.
-
The manufacturing experts (and any other stakeholders) green light the project so the model gets integrated into the live systems. At a certain point, a manufacturing expert notices some inaccuracies, and again sends the data experts back to the drawing board.
-
The manufacturing experts (and any other stakeholders) green light the project so the model gets integrated into the live systems. It stays in the live system until some factors change (there’s a new “ground truth”), and the team, again, has to revisit the model.
Data science is a black box – the technician or manufacturing manager doesn’t know the language of the data expert, so they can only assess the model’s quality when it is packaged up for them and run through simulations. And the decision about whether it works is treated as black-or-white, and knocks the data team back several stages.
In order to bring data science to the factory floor, we need to adopt more than technical solutions: we need organizational change. We need to change the way in which manufacturing experts & data experts work together.
Bring The Manufacturing Experts Closer to the Action
To explain how data science can be better integrated, let’s have a look at some important basics of what happens on a data science project.
“Data Science” can be broken down into two phases: the first phase (“the creation”) where data experts gather, blend, explore data and determine the rules of their model, and the second phase (“the productionization”) where those rules are applied in a real life environment.
Let’s take the simple example of differentiating manufacturing parts based on their colors. In the creation phase, the data experts would write the rules that categorize green machine parts as “G” and yellow parts as “Y.” The productionization of these rules might be the actual sorting of those parts in real time. The “creation” part of the process roughly maps to the “Define” “Measure” and “Analyze” phases of Six Sigma, while the “productionization” roughly maps to the “Improve” and “Control” phases of Six Sigma. The difference is that Six Sigma tools typically expect a clean and structured dataset – something that takes a lot of work at the onset of the project.

Looping the manufacturing experts in on both phases of the data science, increases the likelihood that the project will succeed. Instant feedback also allows the team to iterate and ultimately increase the speed at which they work.
Bringing Manufacturing Experts into Creation
Currently, in the creation phase, data scientists write code. To a manufacturing expert, their computer screens might look like something from the Matrix. Same is true for the folks in information security and legal. The lack of insight into what’s happening in the data expert team significantly slows them down, because they have to use write-ups, lengthy meetings, and email chains to explain why their solution works.
For this reason, some teams are starting to adopt low-code platforms to build dataflows. Programming in a visual tool, enables data experts to explain what they’re building as they’re building it. It also allows non-scripters to join in themselves–working off of blueprints or pre-defined solutions. These environments work by providing drag and drop nodes that perform actions on data. Those nodes are connected to form dataflows that illustrate where data comes from and how it’s manipulated.
KNIME Software, in particular, is an open technology that gives access to any data type from any data source, while supporting all the latest tools and techniques.
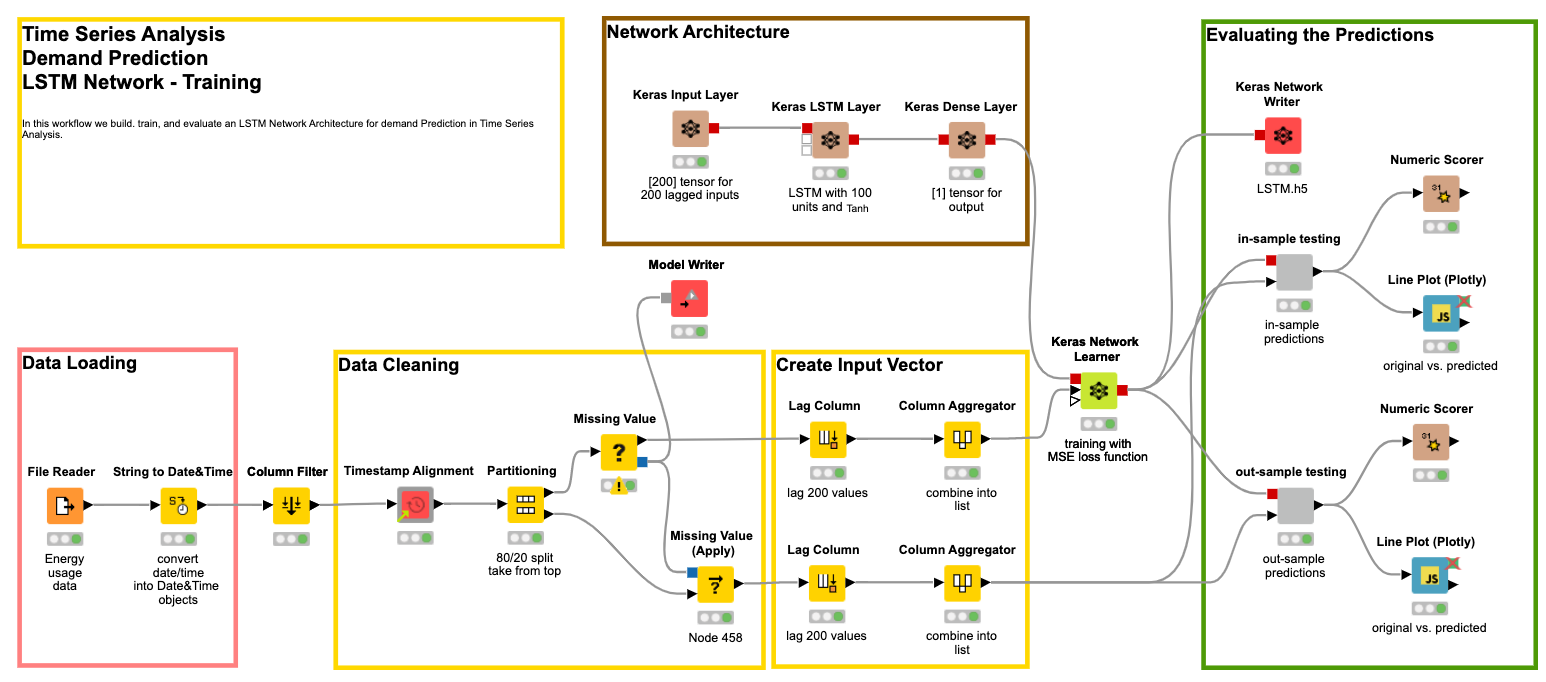
Importantly, it’s much easier to get manufacturing experts to assess the work that a data expert is doing before it’s finished, and for data experts to course correct far before the end of the project.
Bringing Manufacturing Experts into Productionization
Models that are out in the world are imperfect, so manufacturing experts often either:
-
Determine how good is good enough, and;
-
Decide when to override the decision of the model.
There’s a third method, however, that leverages the expertise of manufacturing folks to actually improve the performance of the model.
KNIME allows data experts to use the same low-code tool to put together an interface through which manufacturing managers or operators can interact. In a simple example, below, see how a line manager can alter their workers’ schedules, despite the recommendation of a data science model.
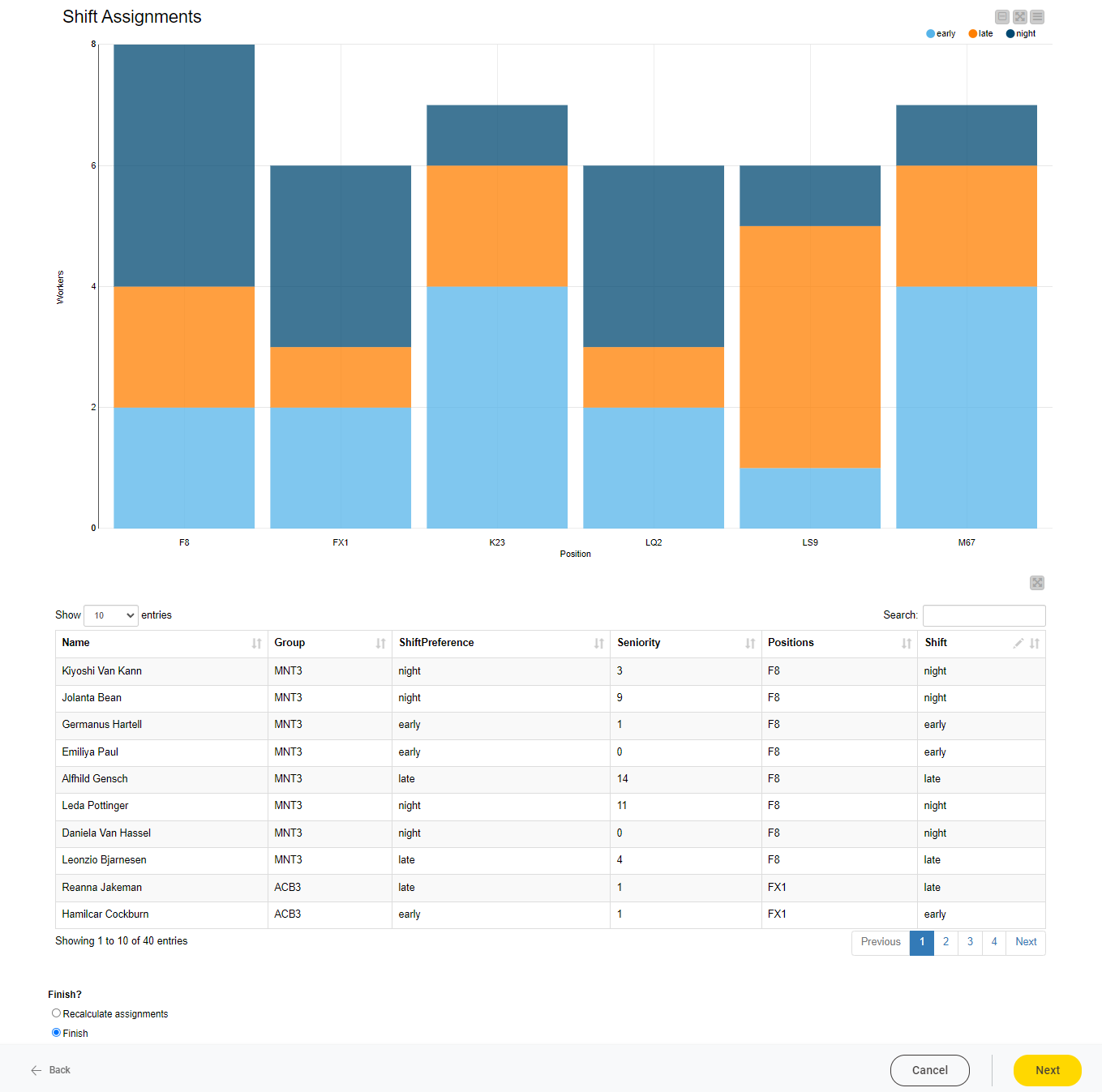
The Guided Analytics interface brings the manufacturing expert into the data science process. Through the application interface, they can be guided to either:
-
Override the decision of the model;
-
Assess the quality of the model, or;
-
Give additional feedback to the model, so it can become more accurate over time.
Power the Factory Floor with Data
When it comes to data science, particularly with respect to advanced methods like AI, leaders often overlook the value of domain expertise. While data experts bring years of mathematical training to the table, operators on the factory floor often bring decades of machinery or product expertise.
Only when both groups can work together, effectively leveraging human and machine intelligence, can manufacturers achieve Industry 4.0 (and beyond).