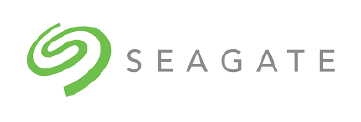
Read success story“KNIME is easy to learn and adopt and is an integral part of our corporate Citizen Data Scientist training program. We’ve had engineers go from complete beginners to analytics practitioners within a few months.”
Allan LukDirector of Data Science and Analytics Business Solutions