Structure-based drug design is a computational chemistry method used to identify new chemical matter based on the structure of proteins involved in diseases. It has become essential for fast and cost-efficient drug discovery. In this blog post, we’ll use the KNIME Analytics Platform to exploit the updated ProteinsPlus web services for automated on-the-fly structural investigations and virtual screening preparations for novel targets of interest. Grab a cup of coffee and click along.
We present you with a workflow for the structure-based analysis of the protein target K-Ras. It is available on KNIME Hub and adjustable to any target of interest. K-Ras is an enzyme involved in the regulation of cell proliferation, and it’s a promising target for cancer treatment. It is referred to as a difficult or “undruggable” target (DOI: 10.1177/2472555217721142), meaning no small molecule modulators have been found, despite intense efforts.
The procedure of target exploration and screening preparation with the KNIME ProteinsPlus components is outlined in Figure 1. It has four steps: extraction of high-quality binding sites, identification of druggable sites, exploration of the binding sites, and preparation of the structures for virtual screening.
Let us guide you through them.
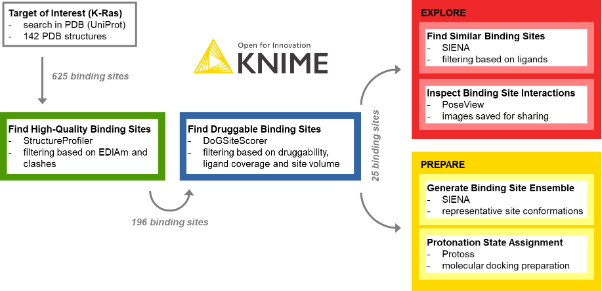
Extracting High-Quality Binding Sites
We start by configuring the Input – StructureProfiler component, providing the PDB IDs for the target of interest and the rule set for evaluating the PDB model’s quality. At the time of the experiment, 142 PDB IDs were reported in the Protein Data Bank for the UniProt Accession Number P01116 of K-Ras. In the meantime, this number increased to 224.
One of the most crucial tasks in structure-based design is the choice of high-quality structures with sufficient electron density support for the structural model, a complete binding site, and the absence of intermolecular clashes. To this end, the StructureProfiler offers crucial information to assess the validity of the available structures. For the 142 target structures of K-Ras from the PDB, we automatically start StructureProfiler jobs with the corresponding component and explore the 625 identified binding sites in the Interactive View of the Table View node (Figure 2). We then use the Rule-based Row Filter node to keep only structures for which a maximum of 10% of the binding site residues are characterized by an EDIA (DOI: 10.1021/acs.jcim.7b00391) below 0.7, and without intermolecular clashes. These criteria can be adjusted when running the analysis for a different target
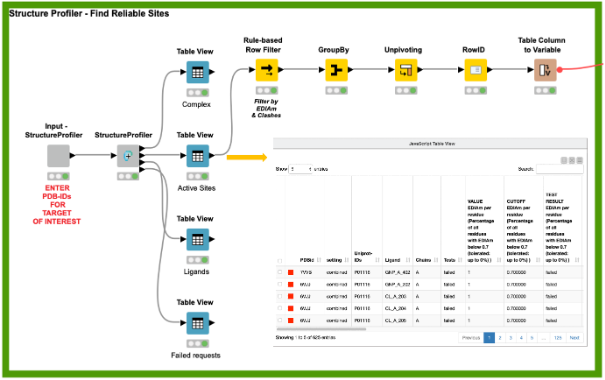
Identifying 25 Druggable Binding Sites for K-Ras
Next we want to learn whether these remaining 196 high-quality binding sites are characterized by a sufficiently high predicted druggability using the DoGSiteScorer component (DOI: 10.1021/ci200454v; see the blue framed annotation box in the workflow). Running this tool for all selected high-quality structures provides us with crucial information, such as geometrical and chemical pocket descriptors and druggability measures. The extraction of sites with a sufficiently high ligand coverage (at least 60% to ensure we can identify and process the correct binding sites), a volume of at least 400 ų, and a druggability score above 0.6 already give us an idea of the relevant binding sites for further screening studies. For K-Ras, we could identify 25 binding sites that fulfill these criteria.
Analyzing and Discovering New Ligand Starting Points
We can now analyze these sites and the interactions of the protein-ligand complexes with the PoseView component (DOI: 10.1093/bioinformatics/btl150,10.1021/ml100164p) and save the corresponding interaction diagrams as images for future reference (Figure 3). Additionally, the PoseView component provides a second output with the binding sites that do not have any interactions with the ligand, like the ligand with the PDB ligand name PG4 in the structure with the PDB ID 5vpy.
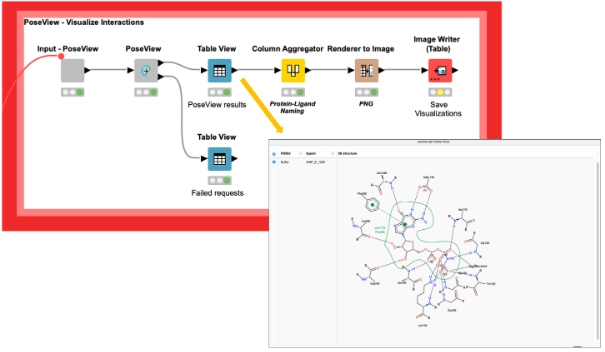
Additionally, we can perform binding site similarity searches with the SIENA component (DOI: 10.1021/acs.jcim.5b00588) for the 25 detected binding sites using SIENA’s flexibility analysis mode. This search might help us develop ideas on how to address the binding sites of interest and assist in exploring the binding characteristics of isoforms. The resulting identified ligands could also provide interesting starting points for pharmacophore searches or molecular docking analyses (SIENA flexibility analysis mode), and can be filtered based on such attributes as active site identity and RMSD to the query.
Note. It is important to emphasize that since all KNIME ProteinsPlus components interact with the ProteinsPlus web services, they use the designated ProteinsPlus input and output format. This means no text processing is necessary when transferring the data from one task to another.
Virtual Screening Preparation – Structure Selection and Protonation
SIENA can be used for more than finding similar sites in complexes with interesting ligands. It might also be applied to generate binding site ensembles for ensemble docking studies.
Using the high-quality druggable binding sites, we can generate ensembles of conformationally diverse binding sites using the screening mode in the SIENA component (Figure 4). In comparison to the web server, we can easily use our workflow to not only generate ensembles for a single binding site of interest but all sites in an automated fashion.
The final decision for a binding site to be further explored can be based on various criteria. In our example, we decided to choose the binding site with the highest number of structural representatives, and generated an ensemble of five conformationally diverse representatives (Figure 4).
We find them by aggregating the PDBid count on the iteration in the GroupBy node, picking the PDBid with the highest count, and then filtering the result table with it using the Reference Row Filter node.
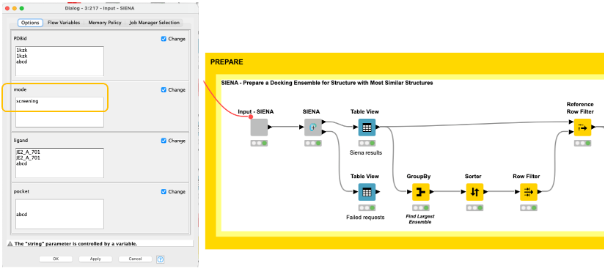
Finally, we prepare five selected binding sites for molecular docking by assigning biologically reasonable protonation states with the Protoss component (DOI: 10.1186/1758-2946-6-12), and then automatically save the prepared structures.
Various extensions and modifications are possible based on the task and the integration of further ProteinsPlus tools in KNIME, e.g. the METALizer for metal geometry analyses (DOI: 10.1093/nar/gkaa235), the EDIA method for crystal structure quality estimation (DOI: 10.1021/acs.jcim.7b00391), the ActivityFinder for small molecule activity searches in databases, and HyPPI for the analysis of the nature of protein-protein interfaces.
Fast Analysis of High-Quality Druggable Binding Sites in KNIME
Our workflow shows that with KNIME, it is straightforward to analyze high-quality, druggable binding sites within minutes. Even the task of binding site ensemble generation and structure preparation for virtual screening can be done within minutes.
The necessary knowledge for using KNIME is easy to acquire by following freely available online examples and learning materials (https://www.knime.com/learning).
We have also demonstrated that with the help of native nodes, such as the Rule-based Row Filter node or the Image Writer node, we can fine-tune and filter the results according to our individual requirements. The results are stored in the corresponding workflows and can be re-evaluated at any time. Finally, as the workflow directly interacts with the ProteinsPlus’ REST API to retrieve the corresponding output, all results can also be visualized in 3D and analyzed using the NGL viewer on the web server (https://proteins.plus). Just replace the PDB IDs in the initial node with the PDB ID of structures for your target of interest and try out the functionality!
Need more help? Contact us via pplus(at)zbh.uni-hamburg.de! We are looking forward to your feedback.