Is #women’s #football different from #men’s? This is the question we asked students at ETH Zürich for a #KNIME student challenge held in November 2023.
If we talk to the average fan on the sofa, yes it is. According to some, women's football is slower, less dynamic, less spectacular, and so on. If we talk to the professionals in the field, no it is not. Or at least any perceptible differences have a lot to do with athletic conditions and professionalization. The questions seemed relevant. Why not have it decided with numbers?
The challenge
The students were given two dataset containing: tens of thousands of events such as passes and shots during matches at the latest #FIFA Men’s and Women’s World Cups. Both are publicly available from data company StatsBomb. Could a machine learning model reliably distinguish between matches played by women or men, but without using athletic features such as top speed? If yes, which would be the most important factors for the prediction? This was the challenge.
51 students signed up, 17 teams were formed, 4 weeks of time was allocated to give the answer. By Friday, 24 November 2023, the submitted projects were evaluated and the winners were proclaimed. The actual award ceremony and the public discussion about their conclusions will take place at the KNIME Data Connect event Soccer Analytics on January 16, 2024, at ETH Zürich.
In general, all participating teams were able to distinguish well between men’s and women’s matches using appropriately trained machine learning models. However, any input features describing physiological and athletic differences had to be ignored, and the real challenge was therefore to argue why they did not creep back in via other features. Discriminant factors were usually related to playing style and technique, especially passing and pressing. However, it was often observed that these differences were so subtle that – although detectable in data – they would be difficult to distinguish by the common spectator’s eye. Most groups used a regression model for the classification task and analyzed its coefficients for feature importance.And the winners are …
The jury was very pleased with the quality of submitted solutions. These often differed substantially in the three dimensions of discriminatory power, workflow design, and justification. The three most original and balanced solutions were ranked as follows.
1st place
Winners are Kshitijaa Jaglan, Gordana Marmulla, Ivana Smokovic, Hadi Sotudeh. This team not only submitted an excellent workflow, but also invested a large amount of time in the review of existing literature on the topic, with the goal of constructing new, possibly more powerful, input features.
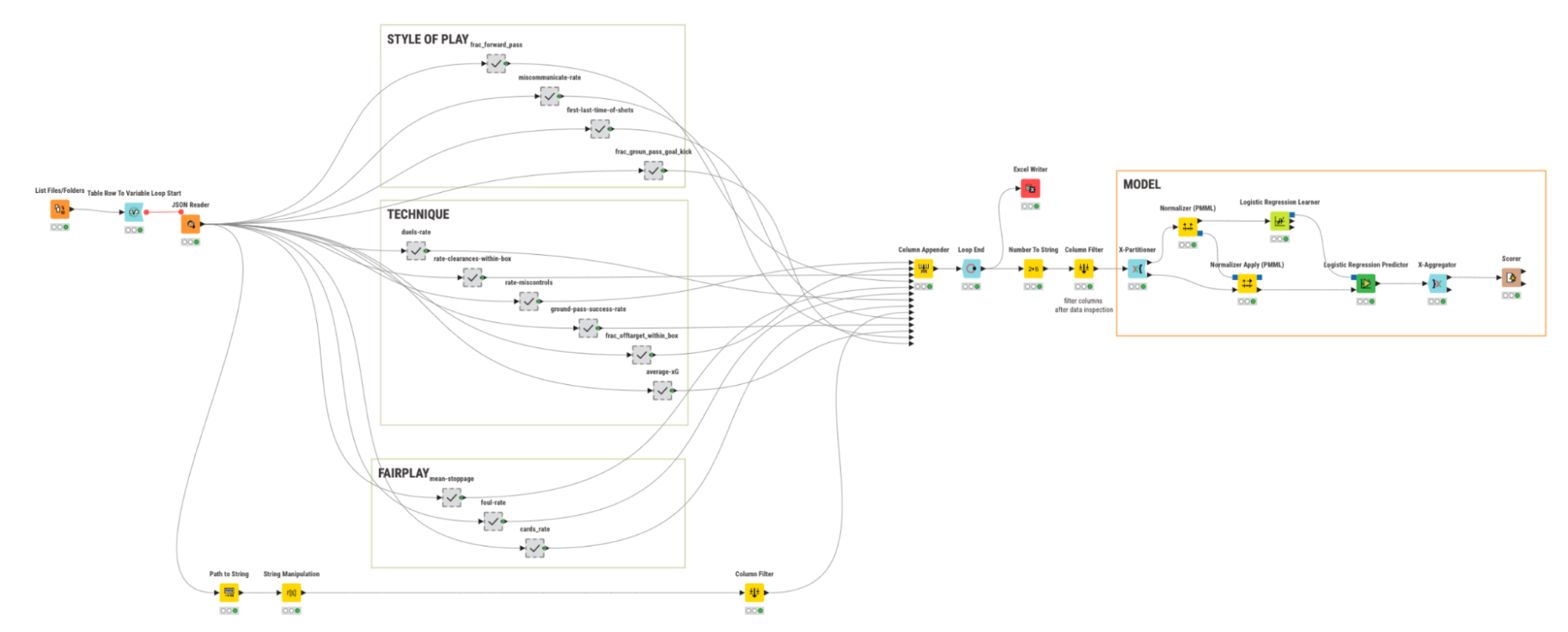
2nd place
As its sole author, Quynh Anh Nguyen impressed the jury with a comprehensive, expertly designed, and well documented workflow.
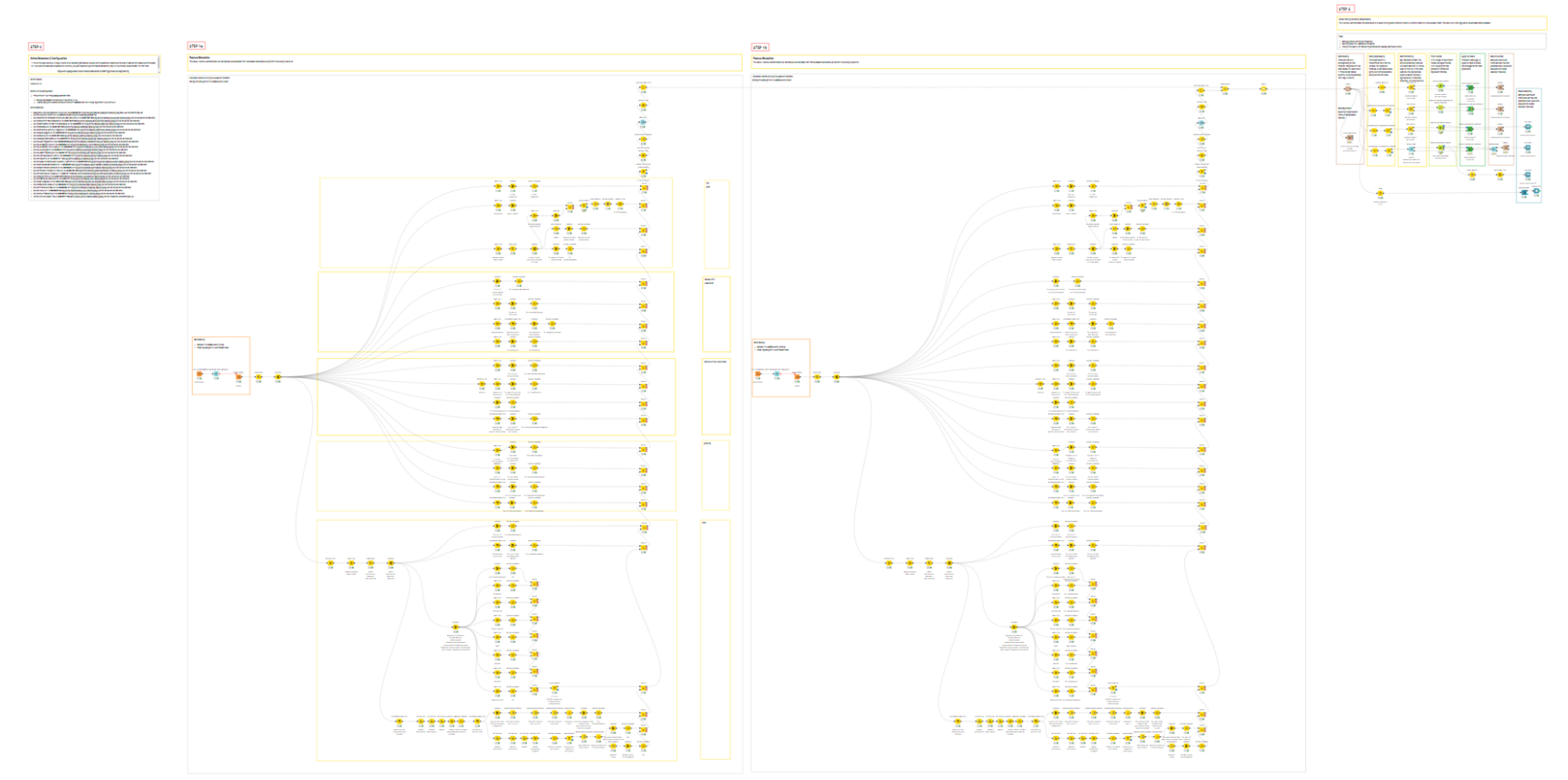
3rd place
Hrvoje Krizic and Simon Zehnde trained a random forest, a logistic regression, and a gradient-boosted tree to distinguish between men’s and women’s matches, and integrated them in a clean workflow.
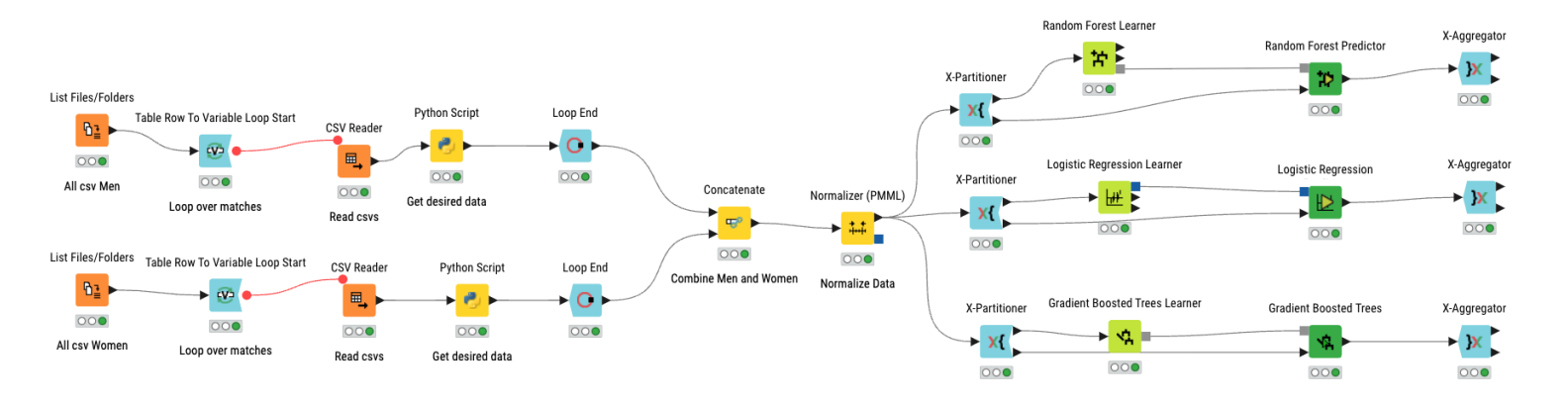
Summary
It turns out it is not so easy to distinguish between the men’s and the women’s game, if you are not allowed to use athletic features, and it is even harder to argue whether this restriction has been observed.
Actually, the topic of this challenge sprouted quite some debate on social media, where soccer fans and data science experts also attempted to generate their own solution. An example is the LinkedIn post by Marcello Pelosi Is Women soccer different from men soccer?
If you are interested to learn about the residual features used by the winning teams, we invite you to the public presentation of their competition entries and award ceremony at the KNIME Data Connect Soccer Analytics event, held on 16 January 2024 at ETH Zürich.
We would like to thank all participants who participated in this first student challenge in soccer analytics. Thanks for your enthusiasm, your curiosity, your time, and of course your willingness to learn something new.
If this has sparked your interest to host a student challenge with KNIME in 2024, please fill out the Student Challenge Application Form.