It’s our natural tendency to resist change, said Newton. And the bigger the object, the greater the tendency to resist.
Despite the fact that leaders recognize the value of upskilling teams across the enterprise, rather than establishing individual pockets of expertise, it’s difficult to build momentum into large-scale upskilling.
But large-scale upskilling is necessary. As automation and AI continue to advance, corporate training in data science and machine learning is critical to ensure a competitive advantage. Companies that invest in enterprise-wide data literacy capabilities and enable employees to interpret and understand data, ask relevant questions of the data, think critically about and analyze data, and use data to make evidence-based decisions, are 3.6x more likely to accelerate their time to market for new products and services and experience strong revenue growth.
Organizations name inadequate training resources as one of culprits slowing down upskilling. Workers state that traditional training resources make it difficult to apply their learned skills in the real world; 94% of business leaders expect employees to simply pick up new skills on the job.
The biggest entry barrier to analytics upskilling is user-friendliness of the tool. Not everyone in the organization will have the same level of technical competence. A tool that makes analytics accessible to even non-technical users through an easy-to-use interface, while not compromising on analytic depth for experts, will garner broader acceptance, and therefore, usage.
By addressing this barrier, organizations can take that first, most critical step towards large-scale upskilling and benefit from the flywheel effect. Here’s how.
The data science upskilling flywheel effect
The upskilling flywheel effect starts when you remove friction by creating a self-serve flow of knowledge. It gains momentum when you enable people of all skill sets to connect and learn from one another.
Key elements of the flywheel are:
- User-friendliness of the tool
- Effortless knowledge-sharing
- Easy delivery of analytical solutions
The easier it is for people to access data science solution starters, training resources, and best practices, the easier it is for more people to learn and develop their own solutions. Momentum builds with each additional solution starter, training resource, and best practice shared by each new user. The flywheel effect is amplified when data insight is deployed and shared easily with business end users as apps and services – making data-driven decisions pervasive across the enterprise.
Let’s see how this works in practice.
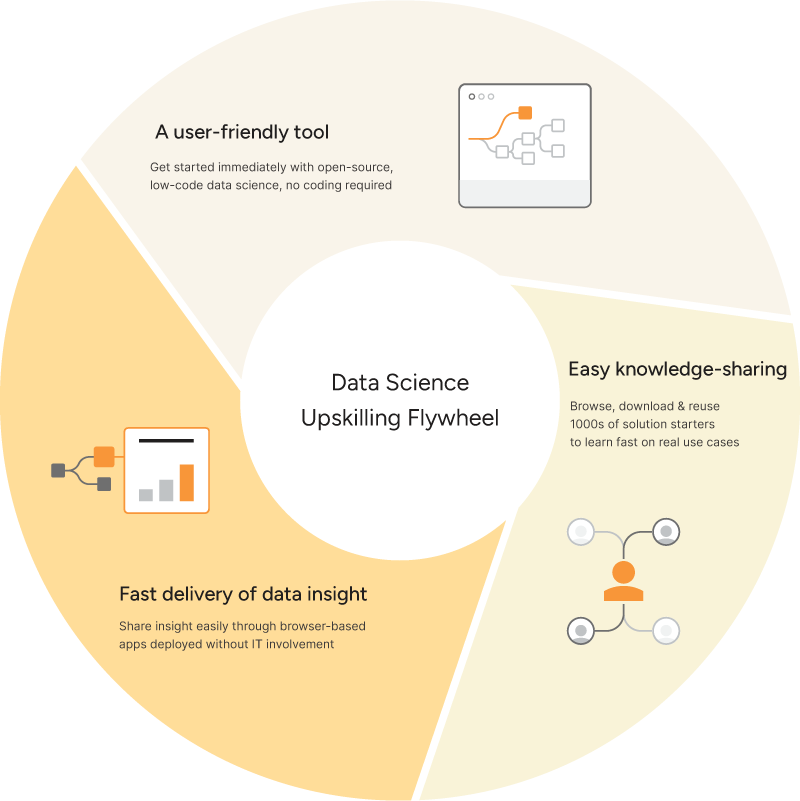
1. An efficient learning curve with a user-friendly, low-code approach
Getting the upskilling flywheel in motion is the hardest. There is a perception that to use a data analytics tool, you need to learn to code. The considerable time it takes to train in traditional coding before anyone can start actually doing anything and seeing a benefit is a real blocker.
We need to find a way for people to lose their fear and learn something new. KNIME is the right software for that.
Christophe Molina, Data Analytics Consultant, Pikairos.
The low-code approach sets the upskilling flywheel in motion
Take Seagate Technology as an example. Seagate is a global manufacturer of data storage technology. Their upskilling initiatives had not been successful despite a keen interest from a range of users to learn more about data engineering and data science. Due to varying levels of experience and ability, upskilling ambitions hadn’t been achieved.
This changed when KNIME, as a no-code/low-code analytics platform, was recognized as a tool that users of all skill levels could engage with to achieve their data analytics needs.
Seagate began upskilling employees, embedding the platform into their high volume manufacturing environment. KNIME’s intuitive interface enabled beginners to focus immediately on learning to apply new data science techniques as opposed to having to first spend time learning to use the tool. Plus, the friction to adoption on the business side was zero, as KNIME Analytics Platform is free and open source: No upfront investment was required.
The learning pace accelerated when teams quickly saw the value of the new skill. In hands-on training, most users were able to get up to speed with KNIME within one or two weeks and see opportunities to apply their newly learned skills to improve day to day tasks. The more they were able to apply their new skills, the faster and more efficiently they learned.
We’ve had engineers go from complete beginners to analytics practitioners in their organizations and implemented KNIME to become part of their daily routines within a few months.
Allan Luk, Director of Data Science and Analytics Business Solutions, Seagate Technology.
2. Effortless knowledge-sharing among teams, departments, or the full organization
After you have instituted a tool that has a short learning curve, make it as easy as possible for teams to work on real use cases. Instead of a blank canvas, give them a head start with access to blueprints related to the tasks they want to solve.
In the next case study, Diaceutics needed a combination of data and business expertise to derive additional data insight.
Diaceutics is a data analytics company in the healthcare space that provides data-driven insights to pharmaceutical companies and biotech from multiple data sources – from lab data to medical claims data, to prescription data, and more. With so much data available and so many details within the data itself, the data analytics team was reliant on the clinical analysts to enrich the data with their medical knowledge, making it possible to reveal previously hidden insight.
This entailed a lot of back and forth between the data and the business experts. It was time-consuming and resulted in translation error. There had to be a better way. They needed an approach that would empower the clinical analysts to add their medical knowledge self-sufficiently.
Access to 1000s of solution starters keeps the upskilling flywheel moving
The clinical analysts were able to learn to use KNIME quickly by accessing multiple solution starters, built and shared by the data analytics team. When faced with new analytics tasks, they could check to see if a colleague had already developed a workflow they could reuse and adapt. They could also browse KNIME Hub for community-built solutions, get started on real use cases quickly, and use new knowledge to build their own solutions.
If more complex or custom functionality was needed, it could be built into so-called components by the data experts and made available to the clinical analysts via component links. This way they could quickly plug new functionality into their workflows.
The biggest wow factors we’ve had come from the clinically minded folks who took the time to learn KNIME and really embed that knowledge and then utilize the underlying power of that data to get the patients on the right drugs.
Scott Morrison, Head of Data Science, Diaceutics in the KNIME Spring Summit 2023 talk, Identifying Clinically Relevant Patient Cohorts
3. Deployment of analytics for pervasive data insight
Now that teams can self-onboard with access to shared solution starters, you can amplify the upskilling flywheel effect by making it easier to deliver data insight across the organization.
Data science upskilling is more than just building sophisticated analysis. It’s also about enabling more people to ask relevant questions of the data, think critically about data, and use data to make evidence-based decisions. But disseminating analytical solutions across the organization can take months, with delays occurring when productionizing has to go through IT.
The Test Systems Product Line at the global automotive supplier, ZF, identified the value of using advanced analytics to optimize end-of-line (EOL) testing. One of the reasons they chose KNIME as their data analytics tool was because they can share their EOL solution easily with multiple factories.
Easy deployment of the solution via a browser-based data app means that the solution can be shared easily with multiple locations without infrastructure expertise.
Gert Jeckel, Senior Manager Data Analytics, ZF Test Systems
Delivery of analytics straight to end users extends the flywheel reach
KNIME software can be used to not just create but also deploy data science solutions without the involvement of IT. This reduces the time between development and deployment considerably.
From the most basic forms to gather data, to interactive dashboards, to the most sophisticated model monitoring, data teams can build and share data apps easily with line-of-business teams via links, or embedded in third party applications.
With data teams able to deliver analysis easily, the upskilling flywheel effect extends its reach. Frontline and non-technical business users are enabled to use analytical solutions via intuitive data apps or REST APIs. They can execute workflows of any complexity and inject their subject-matter expertise without interacting with the underlying workflow.
Free and open source data science dismantles barrier to entry
Data science communities can grow organically and flourish if they are empowered with a common language and the means to quickly share and learn from each other’s solutions.
The cherry on the top is when the data science tool is open source, and free to use. Organizations can make the tool available to all.